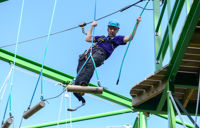
AI Governance - Why does it matter?
Zak Mohammed from Databloom Partners gives us an introduction to Artificial Intelligence (AI), Generative AI and AI Governance
Date: 17th Mar 2025
Author: Zak Mohammed, Databloom Partners
What is AI?
AI, or Artificial Intelligence, refers to technology that is able to perform tasks that mimic human intelligence. This can include skills or outputs such as learning, problem-solving, and decision-making.
It’s likely you’ve interacted with AI without even being aware of it as its use is more woven into our daily lives than we may realise, such as GPS systems determining the route to take, summaries on search engines, and recommendation systems on Netflix and other streaming or social apps.
It does have more obvious applications too. A relevant sporting example would be the use of AI-based platforms to track player movements during games and training sessions. This technology provides insights into fitness levels, tactical positioning, and even injury risks. By leveraging these insights, organisations can make informed decisions that improve both performance and player well-being.
Such applications demonstrate how AI isn’t just a buzzword but a transformative tool capable of addressing real-world challenges.
What is GenAI?
AI experts recognised that, with enough processing power, memory, and a design enabled for more efficient learning and pattern recognition - an AI tool could mimic patterns of language, bring in context, and generate new content.
This same process applies to AI image and video generation, where if enough images of an object, say a cat, are fed to an AI algorithm, it should hypothetically be able to generate an image that looks like a cat. Were this algorithm, instead, trained to be able to identify a cat but not generate an image of a cat, it would then not be classified as Generative AI.
Think of Generative AI (GenAI) as a subset within Artificial Intelligence (AI) with the unique ability to create new things.
Current use in sport
Both within and beyond sport, AI and GenAI hold immense potential. Many tools will use a combination of different types of AI as generative AI will often produce an output after another type has identified what to focus on. Use cases include but are not limited to:
Sport specific |
General (may require human input in conjunction) |
Generating highlights and other short form content from broadcast footage |
Drafting and/or reviewing policy documents (with human input) |
Analysing and providing insights from performance data of players and teams |
Creating high volumes of engaging social media posts |
Using biometric data for injury prediction, prevention and rehabilitation |
Designing promotional materials for events |
Creating highly tailored marketing campaigns for all groups within your audience based on large datasets |
Taking Minutes |
Providing instant training and coaching feedback from video footage to players |
Translation |
Monitoring crowd behaviours to provide crowd safety suggestions during events |
Summarising large reports |
Officiating reviews and assistance |
Skill development in the workplace |
Monitoring of integrity issues |
Customer service chatbots |
Fraud detection and financial management |
|
Recruitment |
|
Supply chain management |
|
Automated journalism |
While AI & GenAI open up exciting possibilities, their use also raises important questions about accuracy, authenticity, and ethical usage, which we’ll explore later in this resource.
Why Should We Be Responsible With AI?
As powerful as AI and GenAI are, they come with significant responsibilities. Without proper oversight, these technologies can lead to unintended consequences that harm individuals, organisations, and society at large.
One major concern is bias. AI systems learn from historical data, and if that data contains biases - whether related to gender, race, socioeconomic status or other characteristics - the resulting outputs will reflect those biases. For example, an AI system used to evaluate athlete recruitment might unfairly favour certain demographics over others, perpetuating inequality within sports.
Another critical issue is misinformation. GenAI models can fabricate convincing yet false narratives, images, or statistics. In sport, a recent example of this came when Apple’s AI tool shared news that darts player Luke Littler had won the final of the World Championship before the match had begun. Whilst this was a minor example with limited consequences, other fabricated news stories may manipulate public opinion about key events and policies. Consider a scenario where a fake announcement about rule changes spreads rapidly online, causing confusion among fans and stakeholders. The impact could range from reputational damage to financial losses.
Privacy is another area of concern. Many AI applications rely on vast amounts of personal data to function effectively. If this data falls into the wrong hands due to poor security measures or a lack of ringfencing within an AI tool, it could result in breaches that compromise sensitive information.
For instance, wearable devices used by athletes often collect biometric data, which, if mishandled, could expose private health details and conditions. Another example could be if a large membership dataset were to be analysed using an AI tool that explicitly states that it will use the data in future models, this would be a large breach of data protection obligations, resulting in vast amounts of data being shared firstly with the AI tool provider and secondly potentially with the wider world using the tool.
These examples underscore why responsibility is paramount when deploying AI. Ensuring fairness, transparency, and accountability isn’t just a moral obligation - it’s essential for maintaining trust and credibility.
Utilised responsibly and with appropriate transparency (internally and externally), AI and GenAI can build trust in an organisation with its ability to tailor to the needs of individuals. The downside of this is that when utilised irresponsibly and opaquely it can quickly unwind goodwill towards an organisation through data breaches and ‘computer says no’ answers when there is insufficient oversight.
How Can We Govern AI Responsibly?
So, how do we ensure that AI is used responsibly? The answer lies in establishing robust frameworks that prioritise ethics, transparency, and inclusivity.
Here are some high-level principles and practices to guide responsible AI governance:
- Ethical Guidelines: Organisations should develop clear ethical guidelines outlining their acceptable uses of AI. These guidelines should address issues like bias mitigation, data privacy, and accountability. For example, you could mandate regular audits of AI systems to ensure compliance with ethical standards.
- Transparency: Transparency builds trust. Stakeholders - including athletes, fans, and sponsors - should have access to understandable explanations of how AI systems work and what data they use. Visualisations can play a crucial role here. For instance, workflows that showcase where data is gathered from and what steps it goes through before a decision is made can help demystify complex processes.
- Human Oversight: While AI can automate many tasks, human judgement remains indispensable. Decisions involving significant consequences - such as disciplinary actions or policy changes - should always involve human review. This ensures that empathy, nuance, and contextual understanding aren’t overlooked.
- Collaboration: Governing AI responsibly requires collaboration across sectors. Sports organisations may look to partner with tech companies, academic institutions, data experts, and regulatory bodies to share best practices and stay updated on emerging trends. Joint initiatives could focus on developing standardised protocols for AI deployment in sports.
- Education and Training: Finally, fostering awareness and expertise is vital. Providing training programs for staff members on AI literacy can empower them to make informed decisions. Workshops, webinars, and interactive modules can serve as effective educational tools.
To illustrate these principles, consider a hypothetical case study:
A national sports federation adopts an AI system to monitor doping violations. To govern this responsibly, the federation implements transparent reporting mechanisms, conducts annual bias audits, and establishes a committee comprising ethicists, legal experts, and former athletes to oversee operations. By doing so, they not only uphold integrity but also set a benchmark for other organisations.
By adhering to ethical guidelines, promoting transparency, and embracing collaboration, sports organisations can harness the benefits of AI while safeguarding against its pitfalls. As we move forward, let us remember that responsible AI isn’t just a technical challenge - it’s a shared commitment to building a better future for everyone involved in sport.
Zak Mohammed is Data Regulations Partner at DataBloom Partners.
Keep an eye out for more content, guidance and tools coming soon to effectively and safely employ AI in your organisation.